Members of the UK Parliament were impressed and somewhat surprised recently by an exchange between the Prime Minister and the leader of the opposition during Prime Minister’s questions.1 The opponents exchanged polite introductions and proceeded to debate the issues of the day in a calm and thoughtful manner, without raising their voice.
The members were in fact watching an exchange with an AI chatbot, programmed to answer questions of the type often raised in the weekly shouting match. Although no insults were traded, the MPs agreed it could well have been mistaken for a genuine exchange. News articles on artificial intelligence (AI) are now a daily occurrence and it is not only through chatbots that AI is making staggering advances.
Machine learning for precision medicine, made easy
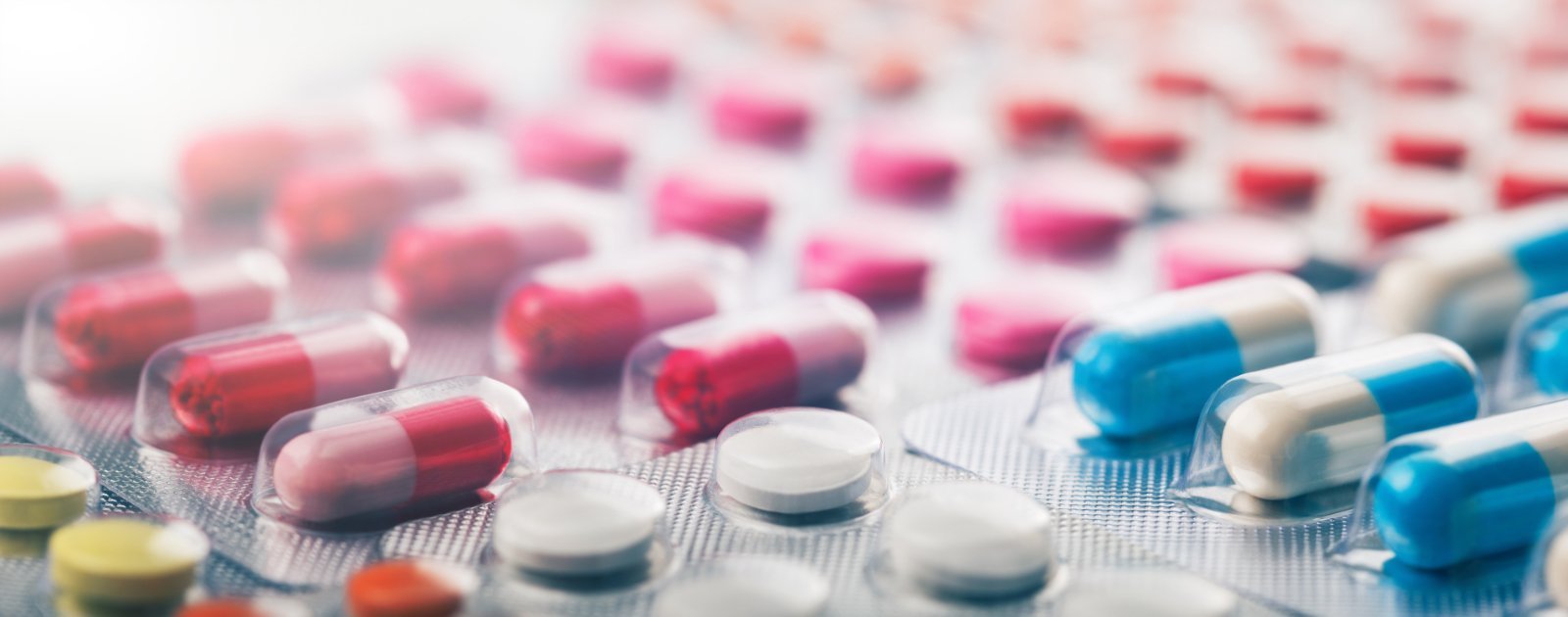
Big data
Many life scientists now routinely generate “big data”- large, diverse datasets, which tend to keep growing. Generating big data is however only part of the challenge. The real value lies in the actionable insights created by analyzing the data. Numerous examples already show the potential of AI to reveal insights not visible by conventional data analysis. Over the next decade, AI has the potential to influence healthcare, finance, and manufacturing.
Medical research was one of the first areas to embrace AI. A room at the Broad Institute in Cambridge, Massachusetts, gives a clue to its attraction. Here, a printed copy of the three billion letters coding for the human genome have been printed (in very small font).2 Sequencing the first human genome cost millions of dollars, years of painstaking lab work and international collaboration to verify the sequences. Now, an entire genome can be sequenced in a matter of hours for around $1,000, making routine sequencing and the generation of enormous amounts of data a possibility. “Big data” had arrived.
Medical researchers recognize that gene sequences are only part of the picture. To properly investigate the causes and possible treatments of disease, we need to consider not only nature (from our DNA) but also nurture; the incredibly complex and data-rich set of environmental and lifestyle factors that may influence our health. Big data has become a lot bigger.
Using human living tissues to test new drugs
At REPROCELL we are interested in the combination of nature and nurture and how it influences drug efficacy. The interest stems from our pursuit of an alternative, if seemingly obvious, approach to the testing of potential new drugs. Rather than testing drugs on mice, or on cell cultures, we conduct tests using donated living tissue samples that are residual to surgery or are not suitable for transplant. Using human tissues from the target patient population might seem obvious, but when we started Biopta in 2002 (now part of the REPROCELL Group) only one other company, Pharmagene, was pursuing a similar approach, based on the use of human data to develop medicines for humans. The initial reception from Pharma was mixed. Some researchers were eager to investigate human biology, others were put off by the more complex picture presented by different responses to drugs in different patients, as opposed to the highly reproducible results obtained from animal models, even if the data from an animal experiment often didn’t predict human responses to a drug. Following the sequencing of the human genome, the industry started to change its view on the value of human data and human tissue testing, and interest turned towards the promise of “personalized medicine”, now more often called “precision medicine”.
Precision medicine aims to tailor treatments to individuals, or to groups of individuals with similar traits. At present, many treatment regimens offer first, second and third-line therapies by a process of trial and error. If you don’t respond well to a first-line therapy (typically the least expensive, generic therapy known to have a clear benefit across many patients) then you are offered a second therapy, and so on, until either the disease is controlled, or treatment options are exhausted.
Precision medicine uses two key sources of information about the individual to predict which therapy is likely to be most effective. These sources relate to nature and nurture. Nature is the genome sequence that makes each of us unique; nurture describes the unique set of experiences that together form our medical history. Increasingly, our medical histories are being captured electronically. Electronic health records are moving from paper-based copies in a hospital filing cabinet to an ever-growing source of big data about the individual stored on multiple databases, including our smartphones, or even on social media. REPROCELL recognized that our tests in human tissues reflected the diverse nature of drug responses found in the patient population, and could be combined with access to deidentified electronic health records and gene sequences of the patients. But the question remained, how could such extensive datasets with diverse clinical and genomic features be harnessed to provide actionable insights that could help develop personalized therapies or guide treatment pathways?
Responders and non-responders to drugs
We were already aware that the human tissues used in the tests at REPROCELL were donated from patients with much more diverse genetic backgrounds than the animals commonly used in scientific research. Most animals used in research labs are either genetically very similar or may even be genetically identical. There are also strict controls on their environment, age, weight, etc., minimizing variation in “nurture”. This is of course quite appropriate for such experiments, but is not always helpful when it comes to predicting the likely response to a new drug in humans. It is perhaps no surprise that most drugs fail during clinical trials due to a lack of efficacy in the target patient population, where the patient group studied in a trial will most often be extremely diverse in age, sex, weight, medical history, lifestyle, and, of course, genetics.
We wondered, could drug responses from human living tissues tested in the lab, which were donated by the target patient population be a bridge between animal tests and clinical trials? Perhaps we could even view our tests as a reflection of the patient population (if enough donor tissues are tested) and try to understand why patients vary in their responses (e.g., so called “responders”, “non-responders” and many patients with a partial response). Testing new drugs in the patient population could help to identify sub-populations of patients most likely to benefit and could reduce high clinical failure rates.
Before we could claim to help, we faced a significant barrier- how could we identify the features that are important in explaining why a patient is a “responder” or “non-responder” to a drug, when faced with big data from DNA sequences and the patient’s medical history?
Using AI to reveal the key features that determine our responses to medicines
The solution to the “big data” problem arrived via a chance encounter at a life sciences conference in Barcelona, organized by DIT and SDI, where a fellow presenter, Edward Pyzer-Knapp from IBM’s AI research department, gave a talk about big data and the uses of AI. After the presentations, I told him about our problem. From our lab tests in human living tissues, we could see the same variation in drug response that is apparent in clinical trials, but it was often difficult to explain why such variations occurred. Edward suggested that a new collaboration between IBM and STFC (the Science and Technology Facilities Council) could be of help, offering access to expertise in the application of AI and machine learning.
We have since have developed a machine learning platform3, Pharmacology-AI, that enables researchers to easily and rapidly find actionable insights about the clinical or genomic features driving drug responses, or other types of functional response, such as a change in a biomarker or clinical measurement.
References
- Kate Whannel. Could a chatbot answer Prime Minister's Questions? BBC News (2022).
- Anne Buboltz. Pages from the first human genome. Broad Institute (2010).
- Gardiner, L et al. Combining explainable machine learning, demographic and multi-omic data to identify precision medicine strategies for inflammatory bowel disease. PLOSOne (2021).