Healthcare data is predicted to expand by 43 percent by 2020, to an incomprehensible level of 2.3 zettabytes. The size of the data is also not the only inevitable issue, it’s the type of data. Eighty percent of it is completely unstructured and mostly unlabelled, meaning organizations will find it increasingly difficult to extract any value or outcomes from the datasets [1].
The accelerated growth of large research cohorts, with links to electronic health record (EHR) data, has uncovered novel indications for existing medications whilst facilitating the discovery of new genetic variants for predicting drug action and response. Establishing patient subpopulations and complicated phenotypes has been made possible by contemporary machine learning and artificial intelligence (AI) approaches, progressing our ability to interpret clinical data [2].
The Exciting Potential of Precision Medicine
The cost of the drug development process has drastically increased over the last decade, as detailed in a recent report by the Tufts Center for the Study of Drug Development[3], which estimates the total cost of developing a drug product to the approval stage to be $2.56 billion. The report also highlighted that an additional $300 million are typically spent on post-approval marketing surveillance bringing the total life cycle costs to $2.87 billion [3]. It should also be noted that drug attrition rates are rising, resulting in huge losses of investment for pharmaceutical companies [4].
Precision Medicine (PM) aims to use pharmacogenomics to identify genetic variations in response to pharmaceuticals. An emphasis on clinical biomarkers clearly sets PM apart from the ‘trial and error’ approach of traditional empirical medicine. Moving away from a ‘one drug for one disease’ strategy, by which it is accepted that a percentage of patients will gain little or no benefit and may experience adverse reactions, PM aims to identify genetic sub-populations and ensure patients receive the treatment most likely to have a therapeutic effect. The potential benefits of a stratified approach to treating disease include a reduction in adverse events, via safe and effective drug delivery and significant financial savings for healthcare systems and pharmaceutical companies, via less wasted medication and a reduction in clinical trial attrition rates [5]
In September of 2018, the First Minister of Scotland, Nicola Sturgeon convened a historic Precision Medicine Summit[6], attended by clinical, industry and academic experts, stating that “Scotland has all of the potential to be a world leader in developing precision medicine”. The summit was co-chaired by the University of Glasgow’s Vice-Principal Professor Dame Anna Dominiczak who explained that PM promises not just improved patient outcomes but savings for the NHS of more than £70 billion [7].
Applying Machine Learning to Large Datasets
Just as the pairing of EHRs with biobank specimens has become a popular approach to healthcare research, emphasis has been placed on the creation of large ‘Population-wide’ datasets to boost the power of bioinformatic analysis [8]. These datasets can then be used to train computer algorithms to produce diagnostic and treatment-based output. This machine learning approach to precision health analytics has the power to translate extensive amounts of data into treatment plans most likely to benefit specific groups of patients.
A recently published study, carried out by researchers at Stanford University[9], reported that their machine learning algorithm is already capable of outperforming human epidemiologists. The newly developed computer system was trained to identify over 10,000 individual traits from unstructured data inputs (histological slides) and was able to more accurately classify specific cancer types than the clinicians.
Additionally, the algorithm will score each slide based on its individual traits alone, as it does not incorporate any professional or scientific over-confidence/complacency. The researchers also highlighted that the algorithm was able to identify visual traits of cancer which were previously unknown when it was left to run without instructions or input from the researchers. This suggests that this type of machine learning algorithm could help us identify and classify new types of cancer, and possibly other diseases, in the future [9].
Machine Learning is Integral to Precision Medicine’s Future
The combination of ever-expanding datasets and widening access to contemporary machine learning and AI systems has led to the development of “deep learning” methods. These can identify unique characteristics from a dataset and, in a similar manner to the algorithm highlighted above, do not need human supervision.
This approach has stoked interest from various groups in industry and academia as they have the computing and technical resources to facilitate these deep learning methods and the capability to employ them on national and international patient cohorts [2].
Large, national cohort studies such as The Million Veteran Program [10] and UK Biobank [11] have the potential to significantly scale up ‘big data’ discoveries and advance the development of PM in global healthcare systems. These studies and others collectively foresee an international cohort of millions of patients with extensive EHR data linked to genomic data and other demographic information, which can be accessed by researchers across the globe.
An assortment of multiple complementary datasets provides the necessary genetic density and sample size to advance the discovery of new drug targets, drug effects and genetic variants [12]. This ‘global biobank’ approach when coupled with modern machine learning methods holds the potential to efficiently and accurately produce personalized treatment plans for individual patients and accelerate the adoption of PM in healthcare.
Accelerate drug discovery and development
The entry of machine learning and AI technologies into the PM picture provides organizations with the opportunity to fully capitalize and capture value in this emerging industry. In a clinical setting, AI can allow clinicians to work more efficiently and improve the accuracy of their diagnoses, which helps to increase the overall productivity and effectiveness of healthcare systems.
At an industry level, machine learning with precision medicine can accelerate the drug discovery and development process to cut costs, reduce errors and gain faster approvals. As the amount of available healthcare data grows exponentially, AI can deploy deep learning methods which overcome the problems associated with large, unstructured datasets to extract clinical outcomes and demonstrate the power of precision medicine.
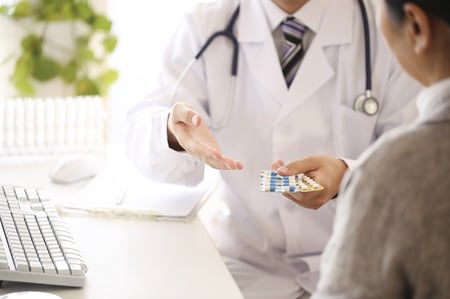
Precision Medicine Services
We are revolutionizing the way medicines are developed and selected for patients.
References
- EMC2. The Digital Universe – Driving Data Growth in Healthcare (2014)
- Denny et al. The Influence of Big (Clinical) Data and Genomics on Precision Medicine and Drug Development. Clinical Pharmacology & Therapeutics 103:3 (2018)
- DiMasi, Grabowski and Hansen. Innovation in the pharmaceutical industry: New estimates of R&D costs. Journal of Health Economics 47 (2016)
- Waring et al. An analysis of the attrition of drug candidates from four major pharmaceutical companies. Nature Reviews Drug Discovery 14:8 (2015)
- Wafi and Mirnezami. Translational–omics: Future potential and current challenges in precision medicine. Methods 151 (2018)
- Scotland could be 'world leader' in precision medicine, says FM. BBC news (2018)
- University of Glasgow. First Minister leads historic summit on Precision Medicine. University news - Archive of news (2018)
- Caenazzo, Tozzo and Borovecki. Ethical governance in biobanks linked to electronic health records. European Review for Medical and Pharmacological Sciences 19:21 (2015)
- Yu et al. Predicting non-small cell lung cancer prognosis by fully automated microscopic pathology image features. Nature Communications 7:1 (2016)
- Gaziano et al. Million Veteran Program: a mega-biobank to study genetic influences on health and disease. Journal of Clinical Epidemiology 70 (2016)
- Sudlow et al. UK Biobank: an open access resource for identifying the causes of a wide range of complex diseases of middle and old age. PLoS Med 12:3 (2015)
- Zatloukal et al. Biobanks in personalized medicine. Expert Review of Precision Medicine and Drug Development 3:4 (2018)