Bias in AI for precision medicine
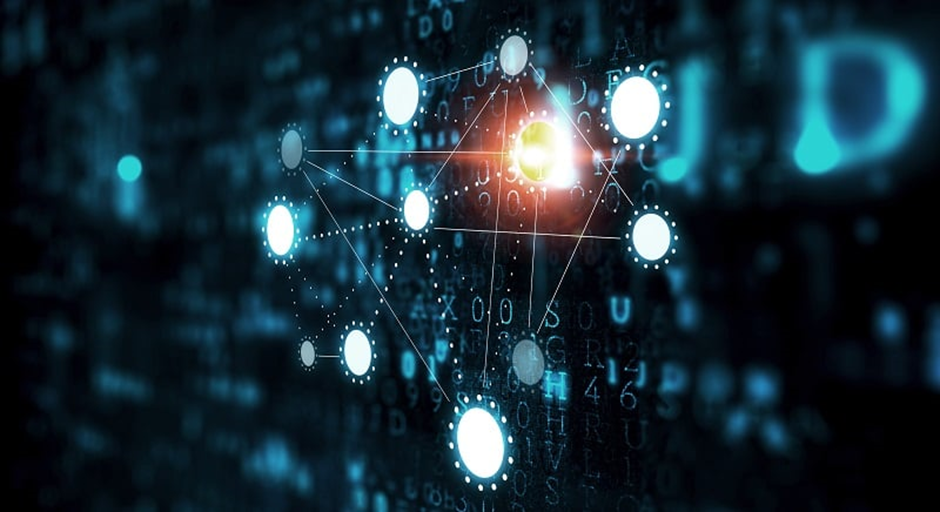
In the world of research and development AI is still in its infancy. Though it is a relatively recent addition to the medical toolbox, AI already offers many advantages. For example, AI can help streamline drug discovery, reducing the cost of developing safe and effective medication. AI may also play a role in precision medicine by creating individualized treatment plans, reducing patients’ exposure to ineffective or unsafe interventions and may improve overall patient outcomes.
This post is the first in a two part series:
- Bias in AI for precision medicine
- Data Privacy and Protection in AI for Precision Medicine
While there are many valuable benefits, there are also significant ethical and regulatory challenges. Bias within the trained algorithms, diversity in data representation and the protection of patient privacy, are some key areas of concern. Looming large over this field is a key question: how can clinicians and researchers exploit the benefits of AI, while avoiding bias and representing the global population? For part one of this topic, we will explore concerns around bias in AI.
Biased datasets can skew outcomes
Without proper and due consideration in the design and delivery of new therapies and diagnostics, there is a risk that biased datasets will skew outcomes towards certain groups. AI systems are not immune to such bias, requiring, by their nature, input data to “train” the system and identify patterns that relate features (whether biological or demographic) to outcomes. Trained AI systems can then predict outcomes, such as a patient’s response to a drug, only when the input features are known. The accuracy of such predictions are therefore highly dependent on the input (training) data used to build the AI algorithms. The training data should represent the patient population, without being skewed towards particular demographics. It is the responsibility of researchers, clinicians, and AI developers to recognize areas sensitive to bias and, when generating training data, take appropriate measures to recognise any inherent bias. Failing to recognise such bias, either during the creation or use of AI systems, could result in the improper treatment and diagnosis of patient groups that should be benefiting from these exciting advancements. There have already been documented instances where marginalized groups have been negatively impacted by the projected bias of AI technology. Concerns have arisen around overall patient risk assessments, the relationship between cost and quality of care and the lack of exploration into sex-specific differences in therapeutic responses to treatment. Two main areas of concern are racial bias and sex-specific bias.
Racial Bias
Black and brown patients’ medical care experiences have been historically compromised because of multiple socioeconomic factors, including unequal access to medical care and treatment. The unequal allocation of funding within the medical and healthcare sectors to those communities has resulted in a lower quality of care and higher mortality rates in black and brown patients3, 5. Some AI algorithms in development and early use appear to already show digital manifestations of a similar racial bias, for example, in the diagnosis of skin lesions3,5. As a consequence of the limited access to quality healthcare in black and brown communities, medical, demographic and “omic” data collected from these groups may be lacking within healthcare systems, which might then be reflected in their under-representation within training datasets used to generate AI algorithms.
Risk scores are a tool used during medical treatment to predict various patient outcomes. There have been specific instances where risk scores were analysed via AI and, although black patients were known to have higher health risks, they would receive predicted risk scores similar to that of healthier white patients, eliminating them from the appropriate care management programs5. Inherent bias in the data used to generate the AI models thus resulted in the recommendation of treatment plans that were not beneficial to the entire patient population. It is critical that AI platforms avoid racial bias in training datasets, as such bias will lead to higher levels of misdiagnosis and over-prioritization of selected patient groups within the AI Algorithm3,5.
Sex-Specific bias
In addition to racial bias, sex-specific biases must also be avoided. There have been instances where the diagnosis of female patients has been based on our understanding of health conditions in males, resulting in female patients being misdiagnosed. This can manifest in a myriad of ways, but an example is in women often receiving too high a dose of medication, as clinical trials are most often conducted primarily in males. A recent study explored sex differences in pharmacokinetics and how that could affect female patient’s reactions to medication6. The results showed that from a review of 86 FDA- approved drugs, 88% showed sex-based adverse drug reactions in female patients with elevated blood concentrations and longer periods of elimination6. For example, Zolpidem, a commonly used sleep medication, was known to remain in the blood of female patients longer than male patients receiving the same dose6. This drug was developed prior to the implementation of the NIH Revitalization Act of 1993, which enforced the inclusion of women and underrepresented minorities in clinical research studies. Yet it was long after the development of the Revitalization Act, (only within the past 10 years) that the FDA implemented a 50% reduction in the dosage of Zolpidem for female patients, due to its impact on alertness the morning after use2.
Disparities such as these occur because clinical research is often focused on data from males, to avoid the complexity involved in considering both sexes as variables. It is commonly thought that studies utilizing females can be more complex to design and interpret because of the variability of female hormone levels1,4. Though these biological differences between males and females may impact scientific study design, the inclusion of both sexes within basic and clinical research would allow a proper evaluation of the way diseases or medications impact the female population. Best practice would be to avoid the dangerous assumption that both male and female subjects will respond in the same way to new therapies, because if female patients are under-represented in clinical research environments, what will that mean for their representation in the training of AI algorithms for precision medicine? It is difficult to envisage how AI algorithms could be beneficial to all if certain groups are under-represented in the data gathering stages.
Why reducing bias is important
If data used to build and train AI algorithms continues to reflect the biases inherent to some current healthcare systems and medical research practices, the same race and sex-specific biases we see in electronic health data will find their way into precision medicine. Ultimately, such a failure to recognise and avoid bias at this early stage in the development of AI will lead to poor diagnosis and treatment of already marginalized groups. It will therefore be vital to expand the collection of training data for AI systems to include the entire patient population and for regulators to provide clear guidance on avoiding any bias that excludes people from access to future AI-based diagnosis and treatment.
References
- Beery AK et al. Sex bias in neuroscience and biomedical research. Neurosis Biobehav Rev 35(3) pp 565-72 (2011).
- Center for Drug Evaluation and Research. FDA approves new label changes and dosing for zolpidem products... S. Food and Drug Administration. (2013).
- Norori N et al. Addressing bias in big data and AI for health care: A call for open science. Patterns(N Y). 8; 2(10) (2021).
- Wizemann TM et al. Exploring the Biological Contributions to Human Health: Does Sex Matter? Washington (DC) Sex differences beyond the reproductive system: National Academies Press (US); (2001).
- Ziad Obermeyer et al. Dissecting racial bias in an algorithm used to manage the health of populations. Science 36 pp 447-453 (2019).
- Zucker, I., Prendergast, B.J. Sex differences in pharmacokinetics predict adverse drug reactions in women. Biol Sex Differ 11, 32 (2020)
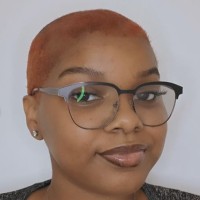
Author
Tiana Hill
Research Technologist at REPROCELL
Tiana joined REPROCELL in 2022 as a research Technologist. She is experienced in academic research, scientific writing, and project management. You can contact Tiana on
LinkedIn.
Subscribe to receive updates from REPROCELL
Stem cell and drug discovery scientists around the world are using our services and products in their preclinical and clinical research.
Subjects we write about
- 3D cell culture
- Cell culture
- Central Lab Services
- Clinical capabilities
- Disease modeling
- Drug discovery
- Gene editing
- Genomic services
- GMP
- Human tissue samples
- Human tissue testing
- IBD
- Life sciences
- Neurons
- Oligonucleotide synthesis
- Precision medicine
- Regenerative medicine
- Respiratory disease
- Skin disease
- Stem cells