Data Privacy and Protection in AI for Precision Medicine
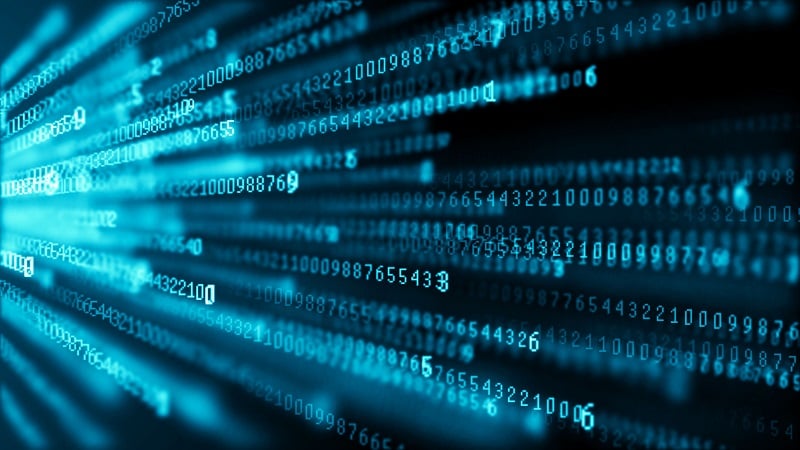
Part one of this blog series, Bias in AI for Precision Medicine discussed the racial and sex-specific bias that has plagued the developing world of artificial intelligence (AI) in precision medicine; diving into why finding ways to rectify that bias is of the utmost importance for ensuring the proper ethical evolution of AI in the world of research and medicine. In addition to those biases data privacy and data protection are further causes of apprehension in the continued transition towards a more AI rich medical research space. Here we highlight some of those concerns and potential solutions.
This post is the second in a two part series:
- Bias in AI for precision medicine
- Data Privacy and Protection in AI for Precision Medicine
For AI to become an integral part of precision medicine, a significant amount of data is needed to train algorithms to accurately predict responses in the target patient population. Access to this data is dependent on the willingness of subjects to provide explicit informed consent. Typically, when undergoing a research project involving human subjects, detailed informed consent documentation is provided in advance of participation, ensuring the patient involved is fully knowledgeable about said research. Various federal institutions enforce rules and regulations globally that should ensure patients and patient health information is protected in any typical research environment involving human subjects or human biospecimen. Ideally, AI research data collection should implement similar processes for protection.
Your questions answered on our machine learning tool for precision medicine
Why data privacy and protection are important
Many still have pressing concerns about data privacy and protection in the space of AI. The anxiety mostly stems from the lack of formal regulation of this new technology, who the main entities are that will have access and control over the personal health information that is being used to develop these algorithms, as well as the overarching risk that patient anonymity could be disrupted with AI.3 How can they be assured that their privacy will continue to be protected throughout its transformation and growth? We are still in the early stages of AI precision medicine development and already experiencing issues with abuse of patient information in the corporate space. Patients involved in any form of research are meant to contribute anonymously and confidentially. Data sharing, though beneficial to the development of AI, could amplify an issue of reidentification within the algorithm. With access to AI algorithms, organizations have been able to utilize public online data collected from patients to re-identify them for their respective projects.3 These possibilities run the risk of exposing the most intimate health information patients have entrusted researchers and clinicians to protect.
Chain of custody (data trails)
This issue of data ownership and access is a common concern for people tapped into the world of AI within healthcare. Since the development of AI algorithms requires large amounts of data, and constant collaboration to fully develop, people are curious about the potential for constant transfer of personal health information data throughout the lifecycle of algorithm development. How will it be monitored, what are the potential risks associated with this open transfer of data? How can we mitigate these risks before they arise, negatively impacting the patients involved?
The prominent companies involved in this technological revolution all seem to have a common goal of growing and developing in the space of AI, sometimes even at the expense of violating the regulations set in place to protect people’s personal health information for the sake of potentially life changing innovation, but the looming question is whether it is worth the risk. In the past 10 years many companies have faced tremendous controversy for exploiting patient information for their own gain .5 Data sharing has occurred between companies and global health entities utilizing millions of patient’s health data for analysis and development of AI healthcare products. Despite light reprimanding and oversight, they have continued to pursue the development of these projects, which shows the relaxed nature of the regulatory bodies in monitoring and rectifying these violations.5
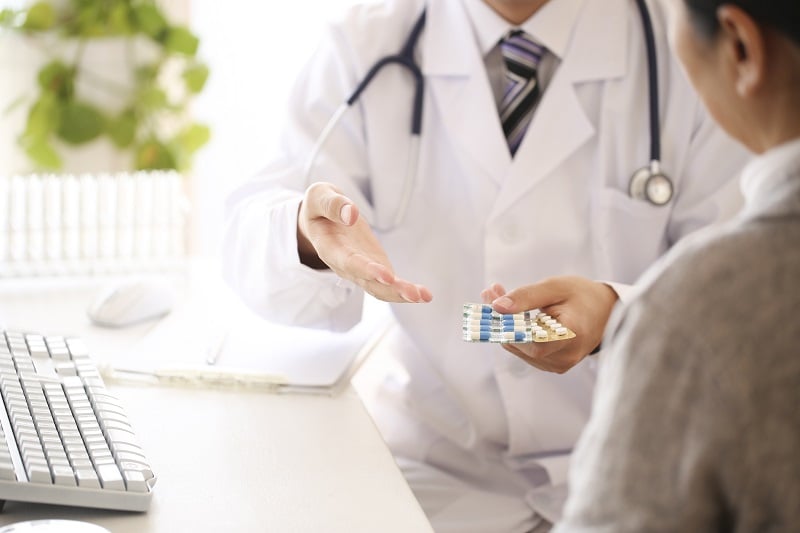
Revolutionizing the way medicines are developed and selected for patients.
Balance between informed consent and innovation
A major aspect of informed consent for patients participating in research studies is the ability to remain anonymous and fully knowledgeable of the potential use of their personal data in research. Issues related to maintaining that confidentiality and anonymity are up for discussion within AI in medical research and precision medicine. Instances of re-identification are a continuing source of worry in the formation of AI algorithms. Data sharing is a major part of the transparency needed to allow researchers to confirm the validity of research findings while also encouraging further development in the space.4 However, re-identification in data sharing has the potential to disrupt the current state of the informed consent process and patient health information protection as we know it.5
Third party access to patient information that has been de-identified is what many researchers have used as a loophole for gaining the appropriate data to develop their health-related projects and products without patient consent .4 Though access to de-identified data is not harmful in and of itself the combination of the sensitive health information and other pieces of demographic analytics can increase the likelihood of reidentifying and pairing patient information directly with specific patients, disrupting the level of confidentiality patients expect to have when providing consent for certain parties to access and utilize their data.2,4
Data governance
Proper data governance can play a major part in soothing the concerns of researchers and patients in the development of AI tools within healthcare and precision medicine. Governance processes can help to ensure the continued protection of patient health information and safety by producing clear, reliable, and fair algorithms for use in medical research.
The foundation for the regulation of data within the space of AI is still in development. No formal federal rules have been put into place. Multiple parties within the field have, however, already begun collating the key principles for implementing AI in the research space, with the FDA developing a plan for monitoring and navigating new AI products and services.1 The FDA proposes AI monitoring under the software as a medical device (SaMD) option. These proposed regulations focus on developing a common practice for good machine learning compliance, monitoring potential modifications, enforcing transparency in algorithmic performance metrics1 to initiate adaptive learning in AI algorithmic development and begin creating guidelines for implementing AI into the medical research space.1
Final thoughts: AI for precision medicine
To maximise the potential of AI in precision medicine we must acknowledge gaps and risks in its development. Those gaps include the overarching and intersecting bias that often exists for patients in healthcare and research, as well as respecting and safeguarding patient data. Not only should such issues be acknowledged, but work needs to be done to implement the proper policies to shift away from any structures that may further perpetuate these real-life biases and continue to protect the people who are doing their part to contribute to major developments. Doing so fosters an increase in trust between patients and industry. The focus should be on reducing the likelihood of creating a space riddled with unequal treatment and misdiagnosis, which could exacerbate existing disparities and mistrust. An inclusive and conscious research environment will produce many more benefits to the world of AI in precision medicine than one that is closed off and fails to represent the entire population. One of the goals of advancing technology in the public health and research space should be to create products by means that improve upon established practices. This starts in the lab environment, as early as the initial collection of data. It also takes place during the recruitment and education of willing participants in research and by diverse patient involvement. Continuous improvement of these processes will mean that we are doing everything we can to develop the best possible version of this potentially life-changing technology.
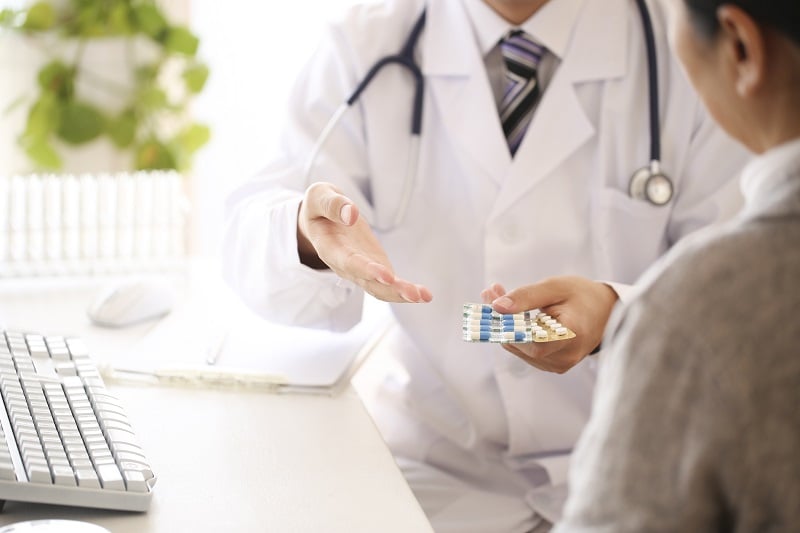
Revolutionizing the way medicines are developed and selected for patients.
References
- Center for Devices and Radiological Health. Artificial Intelligence and machine learning in software. S. Food and Drug Administration. (2021).
2. Kancherla, Jayanth, Re-identification of Health Data through Machine Learning (2020).
3. Murdoch B. Privacy and artificial intelligence: challenges for protecting health information in a new era. BMC Med Ethics 22, 122 (2021).
4.Simon GE, Shortreed SM, Coley RY, Penfold RB, Rossom RC, Waitzfelder BE, Sanchez K, Lynch FL. Assessing and Minimizing Re-identification Risk in Research Data Derived from Health Care Records. EGEMS (Wash DC). 2019
5.Winter JS. AI in healthcare: data governance challenges. J Hosp Manag Health Policy (2021)
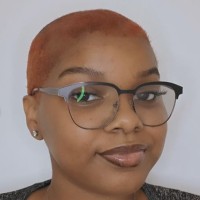
Author
Tiana Hill
Research Technologist at REPROCELL
Tiana joined REPROCELL in 2022 as a research Technologist. She is experienced in academic research, scientific writing, and project management. You can contact Tiana on
LinkedIn.
Subscribe to receive updates from REPROCELL
Stem cell and drug discovery scientists around the world are using REPROCELL’s services and products in their preclinical and clinical research.