The use of animals to predict the risk of adverse effects in humans is a long-standing debate within Pharma and also in society at large. Moreover, safety issues leading to clinical attrition remain a problem and a major financial drain on Pharma.
Previous reviews of the concordance between toxicity in animals and humans have reached conflicting conclusions, partly because different data sets — gathered from either preclinical-clinical tests or findings for approved drugs — were used in the analysis. In this article, we examine the finding of a novel study which sheds light on this hotly debated topic.
Investigating adverse drug effects with Big Data
To determine the role of animal research in adverse drug effects, Mattthew Clark and Thomas Steger-Hartmann have used big data from the Elsevier PharmaPendium database[1] to analyse 3,290 approved drugs and formulations for which 1,637,449 adverse events were reported in humans and animals over the past 70 years [2].
At the heart of their analysis is the calculation of a “likelihood ratio” (LR): a high positive LR (“LR+”) indicates that a drug toxic to animals is likely to be toxic to humans. The LR takes account of not only “True Positives”, but also the number of False Positives and True Negatives to better predict the change in clinical risk when an adverse event is observed in an animal study.
Arguments for and against animal research
Interestingly, the paper supports some of the assertions of previous authors from both sides of the debate. It is clear that many of the preferred animal tests, for example, for the prediction of cardiac arrhythmia, are good predictors of cardiac risk in humans and that both the species selection and endpoints are appropriate based on the pharmacology and high positive likelihood ratio.
For some tests, there is strong concordance because particular endpoints are tested preferentially in certain species and there appears good reason for doing so. Big data approaches might help identify areas of weak concordance, which in turn highlight the lack of pharmacological relevance. In our experience at REPROCELL (Biopta), this reflects the use of human fresh tissue models as filling critical knowledge gaps or helping to understand the risks in translation from animals to humans where the pharmacology is poorly understood.
It is also clear from Clark’s findings and that of previous studies, however, that many tests with a high true positive rate don’t have a high likelihood ratio. In other words, once the number of false positives and true negatives are considered, the use of the animal test as a diagnostic of human adverse effects may not be so high. Some events occur frequently in human and animals, such as erythema, anorexia and liver disorders, but the predictive value of the animal test is low.
Another continuing problem is that of false negatives: the absence of animal toxicity doesn’t necessarily translate to an absence of toxicity in humans.
Summary
Clearly, there remains a need for more predictive models, which truly reflect human biology, to better predict those events that are poorly predicted by animals. At REPROCELL (Biopta), we are continually striving to expand our range of human fresh tissue and 3D models of drug safety and efficacy.
REPROCELL (Biopta) hosts the only catalog of human fresh tissue assays with searchable categories of tissue type, receptor, and endpoints across a range of therapeutic areas which you can access here.
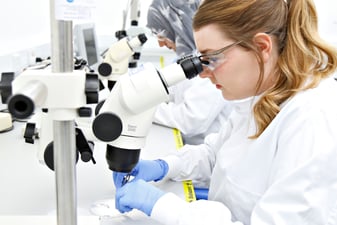
Preclinical and drug discovery CRO
Our contract research services use human living tissues to better predict drug safety and efficacy.
References
- Elsevier PharmaPendium database
- Clark. A big data approach to the concordance of the toxicity of pharmaceuticals in animals and humans. Regulatory Toxicology and Pharmacology (2018)