Outsourcing AI-assisted clinical trial analytics software: what to consider
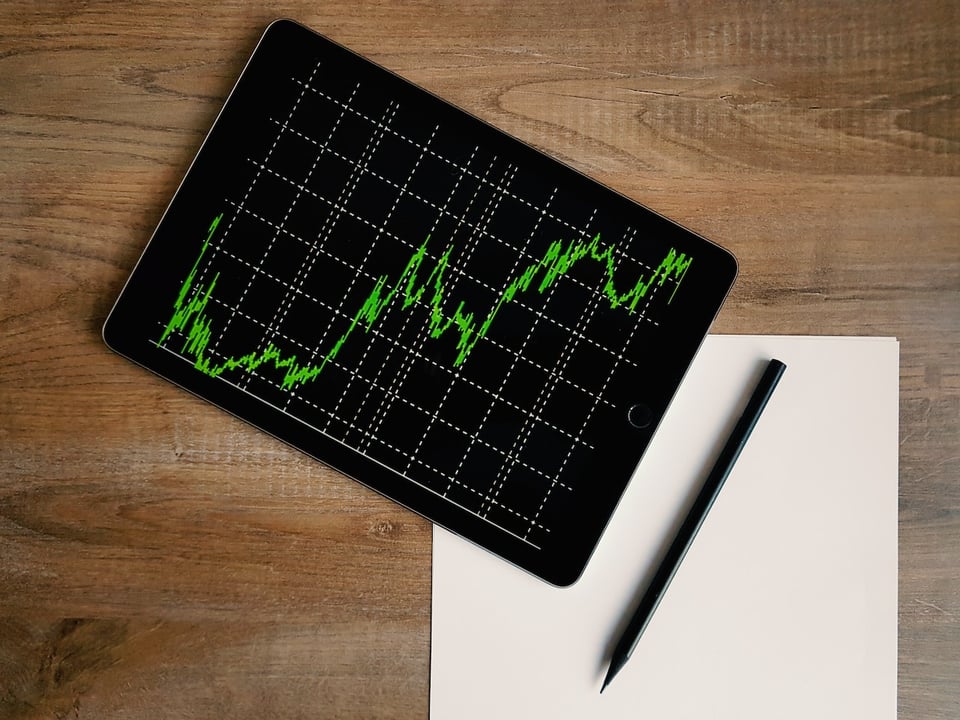
Clinical trial analytics software is used by researchers to collect, store, analyze, and visualize data from clinical trials. These digital platforms can automate complicated analytical processes, improving the efficiency of clinical trials and reducing costs. Artificial intelligence (AI) promises to enhance clinical trial analytics further by automating tasks and providing insights that might otherwise be missed. There are however unique considerations for these machine learning systems that can affect the quality of results generated. In the following article, we will explain the most important considerations when outsourcing AI-assisted clinical trial analytics software.
Security and stability of the system
Data scientists may outsource clinical trial analysis to leverage external expertise or overcome limitations in scalability, time, or resources. Regardless of the reason, it is important to consider the security of the outsourced system to protect patient data and maintain confidentiality. Clinical data should be stored securely by the vendor, so researchers may want to know where the vendor's servers are located, how many backup generators there are, and how quickly any issues can be resolved. It may also be useful to know who can access the clinical trial data and request that anyone with access is named.
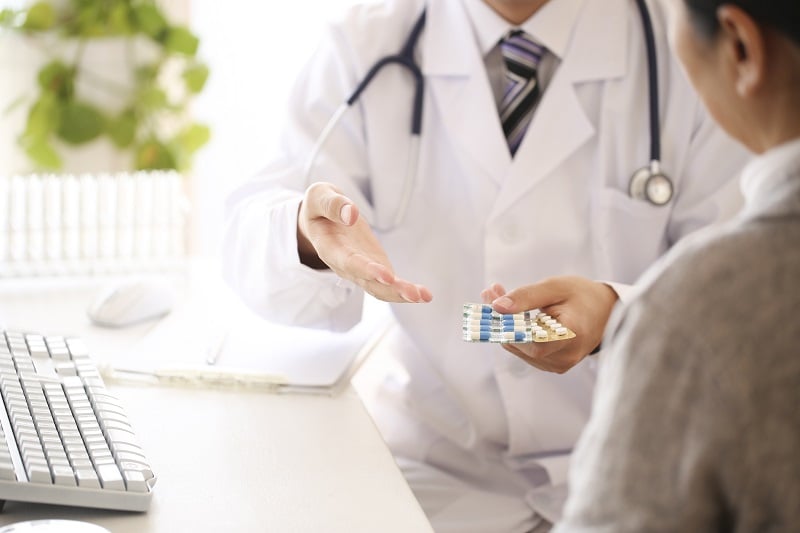
Revolutionizing the way medicines are developed and selected for patients.
Probabilistic versus deterministic models
Due to their inherent randomness and the computational expense of model replication, some machine learning models have limited reproducibility: a cornerstone of clinical research.1 To ensure scientific rigor, researchers should avoid probabilistic machine learning models, as their answers are dependent on context. For example, many of the large language models (e.g., ChatGPT) are probabilistic rather than deterministic, meaning they do not consistently produce the same results. It is therefore advisable to opt for a deterministic machine learning model over a probabilistic one.
Training data
In addition to the type of model used, the data that machine learning models are trained on is key to ensuring the reproducibility of output data.1 If training data is of inadequate quality then predictions made by the model will also be poor, in line with the "garbage in, garbage out" (GIGO) principle. Even if the training data is high quality, models can "drift" from their original set-point over time, and therefore require regular calibration to maintain the accuracy of their predictions.
One way that outsourcing companies can avoid this pitfall is by choosing machine learning software that can be trained on their own data. This ensures that researchers have control over the quality of the training data and can re-train the model themselves when required rather than relying on a pre-trained system.
Machine learning model validation
Unfortunately, the majority of commercially available machine learning models have no peer-reviewed articles supporting their trustworthiness for clinical trial research.1 For most systems, the only "evidence" supporting their use is marketing materials, which do not include details of the models' development and/or performance. To ensure good clinical practice is maintained, researchers can ask whether the development of the vendors' model is supported by peer-reviewed research.
Machine learning model explainability
A final point to consider when outsourcing machine learning-assisted analysis software is whether it is understood how the system makes decisions i.e., model explainability. Machine learning models have a reputation of being a “black box” meaning that their inner logic remains hidden, even to developers.2,3 To build credibility and clinical relevance, the creators of machine learning systems should ensure that their methodologies are transparent, robust, validated, and reproducible.2
Although not yet widely adopted, virtual controlled environments for sharing well-annotated code for models are being developed.2 Quantifying the uncertainty of machine learning systems (which can be influenced by data artifacts, completeness, bias, and accuracy) will also provide systematic frameworks that will improve AI models and build confidence in their decision-making abilities.2
Final thoughts: clinical trial analytics software
When considering outsourcing AI-assisted clinical trial analytics software, several factors must be evaluated. Given the sensitive nature of the data involved, software security and stability are paramount. Comprehensive data cleansing is also crucial for ensuring accurate and reliable results, as high-quality training data is essential to avoid model drift. Other considerations to maintain scientific rigor include whether the model is deterministic or probabilistic, and if its development has been validated through peer-reviewed research.
Lastly, as transparent and robust methodologies are essential for gaining confidence in the system's decision-making abilities, model explainability plays a vital role in building credibility and clinical relevance. By carefully considering these factors, data scientists and managers can make informed decisions when selecting a vendor for AI-assisted clinical trial analysis to ensure the success and integrity of their results.
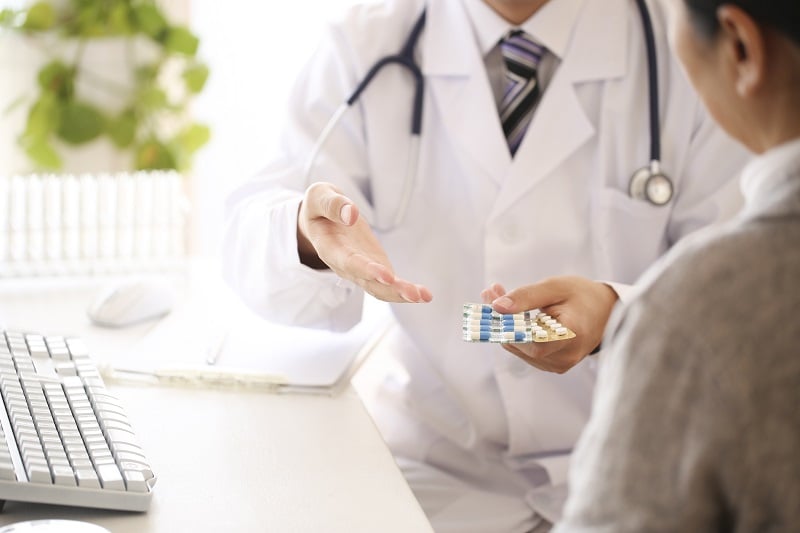
Revolutionizing the way medicines are developed and selected for patients.
References
- Weissler EH et al. The role of machine learning in clinical research: transforming the future of evidence generation. Trials 22:537 (2021).
- Bhinder B et al. Artificial Intelligence in Cancer Research and Precision Medicine. Cancer Discovery 11(4): pp900-915 (2021).
- Vayena E et al. Machine learning in medicine: Addressing the ethical challenges. PLoS Med 15(11): e1002689 (2018).
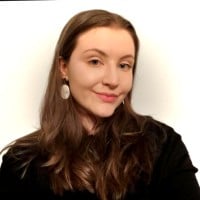
Author
Zara Puckrin, BSc
Zara is a GCU graduate who loves minimalism, marketing, and molecular biology. You can contact her on LinkedIn.
Subscribe to receive updates from REPROCELL
Stem cell and drug discovery scientists around the world are using REPROCELL’s services and products in their preclinical and clinical research.