Clinical trial analytics using AI: everything you need to know
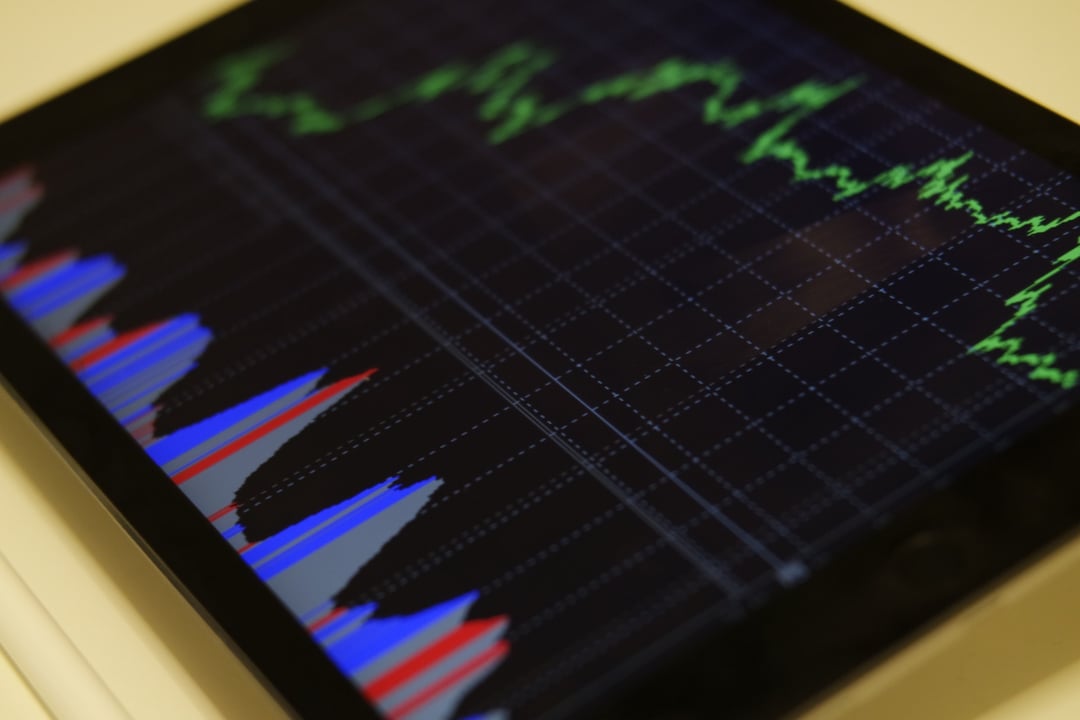
In the ever-evolving landscape of healthcare and drug development, data analytics has emerged as a pivotal force in shaping clinical trials. Clinical trial analytics, a multifaceted discipline, describes the process of deciphering and harnessing the wealth of data accumulated during clinical studies. Its overarching objective is to distill raw data into actionable insights, thereby illuminating pathways to enhance drug efficacy, safety, and patient outcomes.1
In this exploration of clinical trial analytics, we delve into the core principles, the distinctions between primary and secondary analysis, the dynamic interplay of machine learning, and the imperative to address bias and ethical considerations in the pursuit of evidence-based advancements.
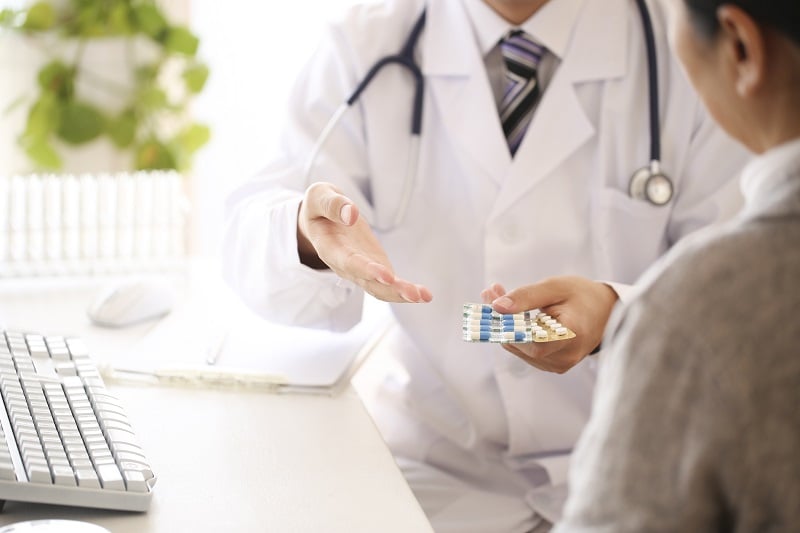
Revolutionizing the way medicines are developed and selected for patients.
What is clinical trial analytics?
Clinical trial analytics describes the process of interpreting and processing the data collected during clinical studies. This transformative process involves the comprehensive analysis of diverse data streams, ranging from electronic health records (EHRs) and laboratory data to wearables and genomic information. The ultimate aim of clinical trial analytics is to create meaningful insights from collected data, identify risks, and improve drug efficiency and safety. While traditionally conducted post-data collection (Figure 1), data managers are increasingly using real-time analysis to inform study design and direction.2
Figure 1: The key processes involved in managing data generated during clinical trials. Data generated during clinical trials comes from a variety of sources including Electronic Data Capture (EDC) systems, laboratories, wearables, and genomic information. Before data can be analyzed effectively, it is usually cleaned to remove any discrepancies and improve its quality before it is analyzed.
Primary analysis versus secondary analysis
There are two different types of data analysis relevant to clinical trials - primary analysis and secondary analysis - and each plays a distinct role. Primary analyses aim to answer the main objective of the clinical trial and are usually pre-specified in the study protocol. They underlie any clinical decisions or recommendations made as a result of the study.3
If investigators have additional questions or objectives they wish to explore they can complete this via secondary analysis. These secondary analyses are supplemental to and/or in support of the primary study objective. They may be pre-specified in the study protocol as supporting objectives, or performed retrospectively by researchers not involved in the original study.3
In a secondary analysis, researchers from academia and industry may wish to:
- perform a sub-group analysis of treatment efficacy/safety
- investigate trial cost-effectiveness
- explore the incidence of adverse drug effects
Depending on the agreement between the sponsor and clinical sites, secondary analyses may be performed for commercial purposes, but access is usually limited for promotional use.3
Intention-to-treat versus per-protocol analysis
There are two different approaches that can be used to analyze data from randomized clinical trials: intention-to-treat (ITT) or per protocol analysis.
Per protocol: During analysis, data may be missing for some enrolled participants when they drop out of the trial or fail to adhere properly to the protocol. Including these data could negatively affect analytical outcomes, so researchers may exclude them, according to the per protocol principle. Per protocol analysis includes data only from participants who fulfil the protocol including adherence to the treatment and outcome assessment. This form of analysis is most likely to highlight the best-case responses to a drug, but because it is possible that the drug itself may have affected adherence to the protocol (yet such data from participants who dropped out is excluded), it could lead to bias.
Intention-to-treat (ITT): As explained above, in randomized control trials (RCT), per protocol analysis can undermine the principles of randomization. It can result in group imbalances and bias, meaning any insights derived from the data can not be generalized to the entire patient population. The FDA, therefore, recommends adhering to the ITT principle by including data from participants who did not adhere to the original protocol or whose data is incomplete.3 In other words, as the name suggests, the results (data) ascribed to the participant are based on the intended initial treatment (the group to which they are originally randomly assigned), and not on the treatment they eventually received (or perhaps not received at all, in some cases). While ITT analysis can underestimate the effectiveness and/or harm of the intervention treatment compared with the control, it also reduces bias and leads to more valid statistical analysis than per-protocol analysis.3
Machine learning for clinical trial analytics
Machine learning models - including random forests, decision trees, and neural networks - are already being used by researchers to accelerate clinical trial analytics. "Machine learning" describes a branch of artificial intelligence (AI) that aims to mimic the way that humans learn (Figure 2). The ability of machine learning to make predictions from unseen data means it has the potential to accelerate several processes involved with clinical trial analysis. For example, clinical researchers could use these technologies to:
- estimate the outcomes of clinical trials under circumstances that do not exist, and use these insights to make clinical trials more efficient
- create "pilot" clinical trials to optimize study design
- predict survival outcomes for patients based on whether they received treatment or not
In these ways, machine learning can suggest treatments and trial designs to help improve patient outcomes. It is hoped that by accelerating clinical trial analytics, machine learning will lead to more effective drug development pipelines and reduce the costs associated with the discovery process.
Figure 2: ML models learn to make predictions from unseen data in a three-step process.
Identifying treatment heterogenicity
In clinical trial analytics, machine learning can also be used to identify differences in the way patient subgroups respond to therapeutics i.e., treatment heterogenicity.4 Knowing which patient subgroups are more responsive to a drug can improve the stratification of subjects for future clinical trials, improve future results, and reduce the risk of clinical attrition.1 For example, where significant efficacy or safety has not been achieved, machine learning can determine whether more significant results could be obtained in specific subgroups. This not only reduces the risk of clinical attrition but could also ensure that novel treatments are prescribed to those who will benefit the most. Great care must be taken however in the conclusions drawn from such post-hoc analysis, as it does not follow good scientific practice by designing a study, conducting a power calculation and then testing a hypothesis. Nonetheless, such post-hoc analysis might generate ideas for new avenues of research and lead to new hypotheses and further clinical trials with greater chances of success.
An even more powerful concept is to use machine learning to reveal insights prior to any clinical trial, by using historical data or data generated from translational preclinical research that reflects the likely variation in the patient population, to help generate hypotheses for clinical trials.
Developing precision medicines
Machine learning models can also take patient stratification a step further by identifying interindividual differences that have the biggest impact on drug response. In a 2023 study, Gardiner et al. used machine learning to discover genetic signatures influencing the response of intestinal tissue from patients with inflammatory bowel disease (IBD) to doramapimod.5 Figure 3 shows how this explainable AI workflow, Pharmacology-AI, used hundreds of data points to identify genetic variations that positively affected treatment outcomes.5 Using machine learning to identify complex correlations in this way could uncover promising avenues for researchers developing precision therapeutics or investigating new drug targets.
Figure 3: Workflow demonstrating how Pharmacology-AI was used to determine the key inter-individual differences influencing drug response so that IBD patients could be better stratified.
Obstacles to machine learning in clinical trial analytics
As discussed, machine learning is already being used to assist data scientists with patient stratification and the development of precision therapeutics. There are however challenges that must be overcome before there can be widespread uptake of AI-assisted clinical trial analytics. Currently, the biggest obstacle is a lack of high-quality training data, which can lead to inaccurate results in accordance with the "garbage in, garbage out" phenomenon. Ultimately, leveraging AI for clinical trial analysis on a large scale will require the improvement of current data management systems used for collecting and storing clinical trial data.
Bias in machine learning predictions
As machine learning techniques become more widely used, it will be critical to ensure that data put forward for analysis represents the entire patient population. This is because machine learning systems can produce biased predictions if their training data misrepresents population subgroups. A real-world example of this is DALL-E 2 (an AI art generator) linking pictures of white men with the prompt "CEO" or "Director" 97% of the time, despite one-third of these positions being held by women.6
Unfortunately, most publicly available datasets in clinical research are limited to specific populations, meaning they either fail to reflect the epidemiology of a demographic or do not contain enough data points.7 For example, the TCGA (the largest biorepository of cancer datasets) is composed primarily of white individuals with European ancestry.4 As race, sex, and socioeconomic factors can influence the outcome of disease and reoccurrence, this can create bias in machine learning systems, which are then unable to accurately generalize their learning to the wider patient populations.4,7 This bias can exacerbate existing health inequalities, meaning that the outputs of the analysis only benefit certain populations.7
Reducing bias by improving data access
In clinical research, bias can be reduced by increasing the ease of data acquisition and the utilization of EHRs.4 EHRs include everything that is known about an individual’s clinical history, making them invaluable for predicting drug response or disease outcomes. Unfortunately, EHRs also remain vastly underutilized in clinical trial analysis, despite being one of the richest sources of patient health and clinical history.4 One reason for the underutilization of EHRs is that they are highly unstructured, meaning that they require exceptional effort to cleanse and curate before they can be used with machine learning.4 Standardization of EHRs through restructuring of these datasets (e.g., the Observational Outcomes Partnership Common Data Model) is one solution that could be implemented, alongside the creation of frameworks that allow effective mining of these datasets.4
To ensure the ethical use of machine learning, it will be important to balance researchers' concerns about data access with data privacy.8 While data privacy laws can exacerbate the lack of representative datasets available to train and test machine learning models, nearly two-thirds of adults in the UK are not comfortable with their personal data being used to improve healthcare.4,7 Using datasets ethically will involve balancing anonymity at the population level while allowing all demographic groups to benefit from the results of machine learning-assisted clinical trial analysis.8 Governments and NGOs will need to play a key role in ensuring the benefits of data sharing are balanced with the right to privacy.
Final thoughts: clinical trial analytics
Clinical trial analytics is crucial for advancing healthcare outcomes globally. The use of machine learning in clinical trial analytics holds promise for accelerating processes, enhancing patient stratification, and advancing precision medicine. Challenges remain, such as the need for high-quality, non-biased training data, which can unleash the potential of machine learning, while balancing privacy concerns. Addressing these challenges in a thoughtful and ethical manner will pave the way for more effective and inclusive clinical trial analytics, ultimately benefiting diverse patient populations and advancing the field of medical research.
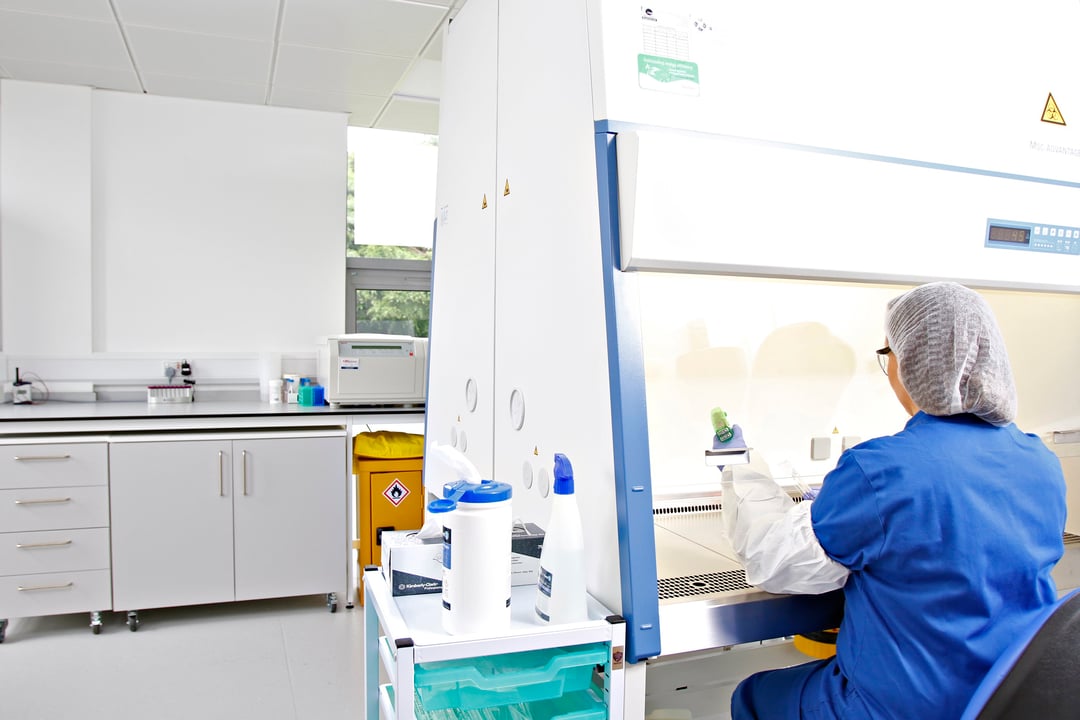
REPROCELL offers comprehensive customised laboratory services for clinical research.
References
- Weissler EH et al. The role of machine learning in clinical research: transforming the future of evidence generation. Trials 22:537 (2021).
- Kunal S et al. Clinical Data Management with Mariam Mirgoli. Clinical Trials Podcast 044 (2022).
- Furberg CD et al. Approaches to data analysis of clinical trials. Progress in Cardiovascular Disease 54:4 pp330-334 (2012).
- Bhinder B et al. Artificial Intelligence in Cancer Research and Precision Medicine. Cancer Discovery 11(4): pp900-915 (2021).
- Gardiner LJ et al. Combining explainable machine learning, demographic and multi-omic data to inform precision medicine strategies for inflammatory bowel disease. PLoS One (2022).
- Luccioni AS et al. Stable bias: Analyzing societal representations in diffusion models. arXiv preprint arXiv:2303.11408 (2023).
- Vayena E et al. Machine learning in medicine: Addressing the ethical challenges. PLoS Med 15(11): e1002689 (2018).
- Hallak R. AI’s Biggest Promise: The Democratization of Precision Medicine. Forbes Technology Council (2023).
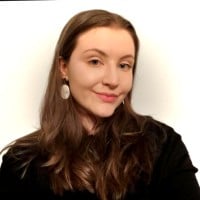
Author
Zara Puckrin, BSc
Zara is a GCU graduate who loves minimalism, marketing, and molecular biology. You can contact her on LinkedIn.
Subscribe to receive updates from REPROCELL
Tagged
Stem cell and drug discovery scientists around the world are using REPROCELL’s services and products in their preclinical and clinical research.