In recent years, artificial intelligence (AI) and machine learning have become integral to the field of pharmacology, revolutionizing how we approach drug discovery, diagnosis, and treatment pathways. As AI's influence grows, so does the necessity for transparency in its decision-making processes. This is where explainable AI comes into play, providing a crucial bridge between advanced computational models and human understanding.
What is Explainable Machine Learning?
Explainable machine learning refers to AI systems that not only provide solutions but also elucidate the reasoning behind their conclusions. As AI begins to play a pivotal role in clinical settings—where decisions can be a matter of life and death—it becomes imperative to understand and trust these decisions. Blindly following AI recommendations without comprehending the underlying rationale is simply not viable. Therefore, explainable AI ensures that the insights and predictions generated by AI are accompanied by clear explanations, enhancing our confidence and trust in these systems.
In the context of drug discovery and medical research, explainable AI is transforming how we approach complex problems. Machine learning models are being used to guide decisions about diagnosis, treatment options, and drug development. However, for pharmaceutical companies, regulatory bodies, and patients, understanding the "how" and "why" behind AI's predictions is as important as the predictions themselves.
Machine learning for precision medicine, made easy.
Introducing SHAP: A Window into Machine Learning Models
One of the most promising tools in the realm of explainable AI is SHAP (SHapley Additive exPlanations). SHAP provides a method to explain individual predictions made by machine learning models, breaking down a prediction into contributions from each input variable. This decomposition allows us to see how different features influence the model's output, providing both local (individual predictions) and global (overall model behavior) interpretability.
How SHAP Works
For each feature in a dataset, SHAP assigns an importance value, indicating its contribution to the model's prediction. In simpler terms, SHAP values show how much each feature contributes to pushing the prediction (of the outcome of interest) higher or lower. These contributions can be summed to reach a base value (the average prediction in the dataset) to reconstruct the model's prediction.
For example, in a binary classification problem (e.g., predicting whether a tumor is malignant or benign), a machine learning model outputs a score between 0 and 1. This score, when compared against a threshold (commonly 0.5), determines the final prediction. SHAP values help explain this score by attributing parts of the score to different input features, giving us insight into the model's decision process.
Practical Applications of SHAP in Drug Discovery
SHAP's ability to quantify feature importance is incredibly valuable in drug discovery and pharmacology. Here’s how:
- Ranking and Weighting of Features: By ranking and quantifying the importance of each feature to the overall response (e.g. a drug response or a clinical outcome), the key factors underpinning a response can be easily identified.
- Modelling of Responses: SHAP makes it easier to predict responses should key features be altered. For example, by selecting patient groups with particular genotypes or demographic features and predicting the response for the chosen group.
- Model Validation: By revealing how a model leverages different features, SHAP allows researchers to validate that the model is making decisions based on scientifically sound principles. For example, if a model predicting drug efficacy is found to heavily rely on irrelevant features, it can signal potential issues in the data or model design.
- Regulatory Compliance: In regulatory settings, there's often a "right to explanation" requirement, where stakeholders need to understand the basis of automated decisions. SHAP helps satisfy these requirements by providing transparent and interpretable outputs.
- Identifying Data Issues: SHAP can uncover unexpected relationships in the data that may indicate problems, such as data leakage. For example, if a non-causal feature shows a strong influence on the prediction, it might suggest that the model has picked up on patterns that should not exist, prompting a deeper investigation into the dataset.
Communicating SHAP Results
While SHAP offers powerful insights, it's crucial to communicate these findings effectively to stakeholders. This involves:
- Simplifying complex outputs into clear, actionable insights.
- Highlighting the main drivers behind model predictions.
- Being transparent about the limitations and assumptions inherent in the SHAP methodology.
Conclusion
Explainable AI, particularly through tools like SHAP, is reshaping the landscape of precision medicine and drug discovery. By providing transparency and clarity in machine learning models, SHAP ensures that AI-driven insights are not only powerful but also trustworthy. As we continue to integrate AI into critical medical decision-making processes, the importance of explainable AI cannot be overstated. It is the key to unlocking the full potential of AI in drug discovery and medical diagnosis or treatment, ensuring that we can confidently rely on these advanced technologies to improve patient outcomes and drive innovation in the pharmaceutical industry.
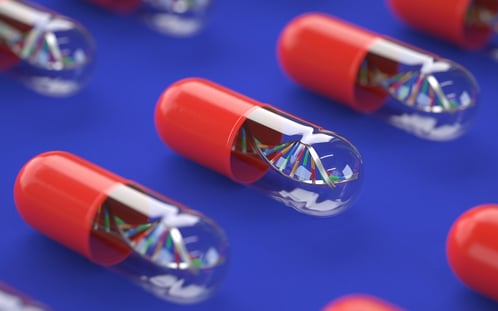